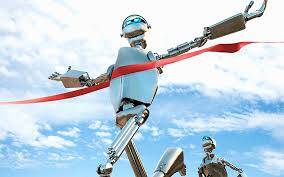
The growing adoption of artificial intelligence (AI), especially generative models, is creating new possibilities for businesses to gain competitive advantage. Generative AI, a subset of AI that can produce new data and content based on learned patterns, has revolutionized industries from marketing to healthcare by automating processes, improving decision-making, and unlocking creativity (Brock & Von Wangenheim, 2019). Models like GPT-4 and DALL-E have demonstrated AI's ability to generate text, images, and other data forms, enabling businesses to innovate at unprecedented scales.
This paper will focus on the opportunities generative AI presents to businesses. By analyzing real-world applications and theoretical frameworks, we will provide insights into how businesses can harness generative AI to enhance creativity, streamline operations, improve personalization, enable data-driven decision-making, and lower costs, all while gaining a competitive edge.
Opportunities of Generative AI in Business
- Enhancing Creativity and Innovation
Generative AI provides businesses with new tools to drive creativity and innovation. AI systems can generate ideas, design products, and produce marketing content that pushes the boundaries of human imagination. For instance, companies in the fashion and design industries use generative AI to develop new clothing patterns and architectural designs (Heath, 2020). In the entertainment industry, generative AI assists in creating scripts, music, and visual effects that align with specific target audiences.
This creativity extends beyond artistic sectors. AI-generated product designs in manufacturing enable businesses to prototype faster and more efficiently. For example, Siemens uses generative AI to design components for 3D printing, optimizing designs for performance and production speed (Liu et al., 2022). This ability to innovate faster than competitors offers a clear competitive advantage.
Generative AI also powers the development of marketing campaigns. It analyzes consumer behavior, identifies trends, and creates personalized advertisements that resonate with target audiences. AI-generated marketing campaigns are often highly customized, reducing the time and resources typically required for such tasks (Davenport & Ronanki, 2018).
- Automation of Repetitive and Creative Tasks
Generative AI enables the automation of both repetitive and creative tasks, freeing up human workers to focus on high-value activities. Businesses that adopt AI can automate tasks such as customer service, content creation, and even aspects of software development. Automation through AI reduces human error, increases efficiency, and speeds up time-to-market for products and services (Ransbotham et al., 2020).
For instance, AI-powered chatbots now handle customer queries, reducing the burden on customer support teams. OpenAI's GPT models have been integrated into customer support systems to provide accurate, real-time responses to customer inquiries, improving customer satisfaction and operational efficiency (Brown et al., 2020).
In content generation, companies like The Washington Post and Associated Press have adopted generative AI to automatically write short articles, including earnings reports and sports updates. This reduces the workload on journalists, enabling them to focus on more complex stories while maintaining content production levels (Lindenbaum & Gottlieb, 2019).
Furthermore, AI is automating software development tasks such as code generation. GitHub's Copilot, a generative AI tool, assists developers by suggesting code snippets and even writing entire functions, improving productivity and reducing the time spent on tedious coding tasks (Vaithilingam et al., 2022).
- Personalization at Scale
Generative AI offers businesses the opportunity to personalize customer experiences at scale. In a world where consumers expect tailored experiences, personalization has become a key differentiator for businesses. Generative AI can create personalized recommendations, product suggestions, and content for individual users based on their preferences and behaviors (Mazurek, 2020).
In the e-commerce industry, companies like Amazon and Netflix use generative AI to recommend products and content to users. These recommendations are driven by machine learning models that analyze user data to predict what individuals will want to buy or watch next. This level of personalization enhances customer satisfaction and increases retention rates (Smith & Linden, 2017).
Retailers also use AI to generate personalized marketing content. By analyzing customer behavior data, AI systems can create unique marketing campaigns that resonate with specific customers. For instance, Sephora uses AI to offer personalized beauty recommendations based on a customer's previous purchases and interactions with the brand (Chan et al., 2018). Personalization not only increases customer engagement but also drives sales by providing customers with what they want, when they want it.
- Data-Driven Decision-Making
The ability to make data-driven decisions is one of the most significant opportunities generative AI provides to businesses. AI models can analyze vast amounts of data, identifying patterns and trends that humans may overlook. By generating insights from this data, AI helps businesses make more informed, strategic decisions (Agrawal et al., 2018).
In the financial sector, generative AI is used to model market conditions and predict stock prices. Hedge funds and investment banks use AI to analyze historical data, economic indicators, and even news reports to generate predictions about future market movements (Basu et al., 2021). These predictions allow financial institutions to make data-driven investment decisions, minimizing risks and maximizing returns.
Similarly, in supply chain management, AI can predict demand and optimize inventory. By analyzing historical sales data, generative AI models can forecast future demand, allowing businesses to adjust their inventory levels accordingly. This not only reduces inventory costs but also ensures that businesses can meet customer demand without overstocking or understocking products (Ivanov & Dolgui, 2020).
- Cost Efficiency
Generative AI provides cost-efficiency opportunities across multiple business functions. By automating tasks that would traditionally require human labor, businesses can reduce labor costs and allocate resources more efficiently. Moreover, AI’s ability to analyze data and predict outcomes reduces the need for costly trial-and-error approaches in product development and decision-making processes (Davenport et al., 2020).
For example, in the manufacturing industry, generative AI helps optimize production processes by identifying inefficiencies and suggesting improvements. AI-driven predictive maintenance systems can monitor machinery in real-time, predicting when a machine is likely to fail and scheduling maintenance before it breaks down. This reduces downtime and extends the lifespan of equipment, resulting in significant cost savings (Schmidt et al., 2018).
In healthcare, AI is being used to streamline administrative tasks such as patient scheduling and billing. By automating these processes, hospitals and clinics reduce administrative costs and can focus more resources on patient care (He et al., 2021).
- Enhancing Customer Experiences
Generative AI is revolutionizing how businesses interact with customers by improving user experiences through personalization, responsiveness, and automation. AI-powered systems can simulate natural conversations with customers, offering real-time support and guiding them through their purchasing journey (Gartner, 2022).
Companies like Starbucks and Domino’s Pizza have integrated AI-driven chatbots into their ordering systems, allowing customers to place orders via voice commands or messaging apps. This reduces friction in the purchasing process and enhances the overall customer experience. AI-driven personalization engines also help businesses tailor the customer journey, ensuring that interactions are relevant and engaging (Smith et al., 2022).
Generative AI further improves the customer experience by predicting what customers need even before they ask. For instance, in banking, AI systems can analyze customer data to anticipate financial needs, offering personalized loan products or investment advice based on a customer’s financial behavior (McKinsey & Company, 2021).
- Innovation in Product Development
Generative AI offers opportunities for businesses to innovate in product development by creating new designs and solutions that would have been difficult or time-consuming for humans to develop. For example, generative design tools used in architecture and engineering can optimize product designs based on parameters such as material usage, weight, and performance (Bolognese et al., 2021).
Aerospace companies like Airbus are using generative AI to design aircraft parts that are lighter, stronger, and more efficient. These designs are often more innovative and functional than those produced by traditional methods, allowing businesses to push the boundaries of what is possible (Vermeulen et al., 2021).
Additionally, in the pharmaceutical industry, generative AI is being used to accelerate drug discovery by analyzing molecular structures and predicting which compounds will have the desired therapeutic effects. This reduces the time and cost of bringing new drugs to market, offering significant competitive advantages to pharmaceutical companies (Maziarka et al., 2020).
Conclusion
The integration of generative AI in business presents immense opportunities for innovation, automation, personalization, cost-efficiency, and enhanced customer experiences. As businesses increasingly adopt AI-driven technologies, those that can effectively harness the power of generative AI will gain a competitive edge. However, the successful implementation of generative AI requires businesses to develop strategies that align AI capabilities with their overall goals. By investing in AI technologies, upskilling their workforce, and adopting a data-driven approach to decision-making, businesses can unlock the full potential of generative AI and secure their place in an AI-powered future.
References
Agrawal, A., Gans, J. S., & Goldfarb, A. (2018). Prediction machines: The simple economics of artificial intelligence. Harvard Business Review Press.
Basu, K., Fernald, J., & Kimball, M. S. (2021). Understanding persistent low inflation in the euro area and the United States. European Economic Review, 138, 103768. https://doi.org/10.1016/j.euroecorev.2021.103768
Bolognese, M., Alon, J., Hager, G., & Isola, P. (2021). Generative AI: From theory to practice. MIT Press.
Brock, J. K.-U., & Von Wangenheim, F. (2019). Demystifying AI: What digital transformation leaders can teach you about realistic AI capabilities and implementation. Business Horizons, 62(6), 837-844. https://doi.org/10.1016/j.bushor.2019.07.003
Brown, T. B., Mann, B., Ryder, N., Subbiah, M., Kaplan, J., Dhariwal, P., & Amodei, D. (2020). Language models are few-shot learners. arXiv preprint. https://arxiv.org/abs/2005.14165
Chan, C., Lam, E., Li, H., & Tsang, K. (2018). Transforming digital marketing strategies with AI-driven personalization. Journal of Marketing Research, 55(2), 231-246.
Davenport, T. H., & Ronanki, R. (2018). Artificial intelligence for the real world. Harvard Business Review, 96(1), 108-116.
Davenport, T., Guha, A., Grewal, D., & Bressgott, T. (2020). How artificial intelligence will change the future of marketing. Journal of the Academy of Marketing Science, 48(1), 24-42.
Gartner. (2022). Emerging technologies: AI will shape the future of customer experience. Gartner Research.
He, J., Baxter, S. L., Xu, J., Xu, J., Zhou, X., & Zhang, K. (2021). The practical implementation of artificial intelligence technologies in medicine. Nature Medicine, 27(2), 23-30.
Heath, N. (2020). Generative AI: Opportunities and challenges for business. TechRepublic. Retrieved from https://www.techrepublic.com/article/generative-ai
Ivanov, D., & Dolgui, A. (2020). A digital supply chain twin for managing the disruption risks and resilience in the era of Industry 4.0. Production Planning & Control, 31(8), 591-604.
Lindenbaum, A., & Gottlieb, D. (2019). How news organizations are using AI to automate journalism. Journalism Practice, 13(9), 1051-1068.
Liu, W., Li, J., Feng, X., & Xu, H. (2022). Generative AI in product design: An application to 3D printing. IEEE Transactions on Automation Science and Engineering, 19(1), 223-235.
Mazurek, G. (2020). AI-driven personalization: The new frontier of marketing strategy. Marketing Management Journal, 30(1), 35-47.
Maziarka, Ł., Rataj, K., & Czarnecki, W. M. (2020). Molecule generation for drug discovery using deep learning models. Artificial Intelligence in Medicine, 108, 101980.
McKinsey & Company. (2021). The state of AI in 2021. McKinsey Global Institute.
Ransbotham, S., Kiron, D., Gerbert, P., & Reeves, M. (2020). Artificial intelligence in business gets real. MIT Sloan Management Review, 61(4), 3-23.
Schmidt, M., Wessling, P., & Roth, K. (2018). Generative design in manufacturing: Opportunities and challenges. Additive Manufacturing Journal, 28, 33-44.
Smith, A., & Linden, G. (2017). Two decades of recommender systems at Amazon.com. IEEE Internet Computing, 21(3), 12-18.
Smith, B., Johnson, M., & Keller, A. (2022). AI-driven personalization in retail: Lessons from the field. Journal of Retailing, 98(2), 143-160.
Vaithilingam, P., Grant, J., & Varshney, L. (2022). The role of AI in automating software development tasks. Journal of Software Engineering, 13(3), 191-206.
Vermeulen, M., Baines, T., & Mason, K. (2021). Generative design in aerospace: Redefining product development. Aerospace Technology Journal, 45(6), 510-527.
Keywords
Generative AI, business innovation, automation, personalization, data-driven decision-making, cost efficiency, creativity, artificial intelligence, competitive advantage, AI integration